Unlocking the Power of Image Datasets for Classification
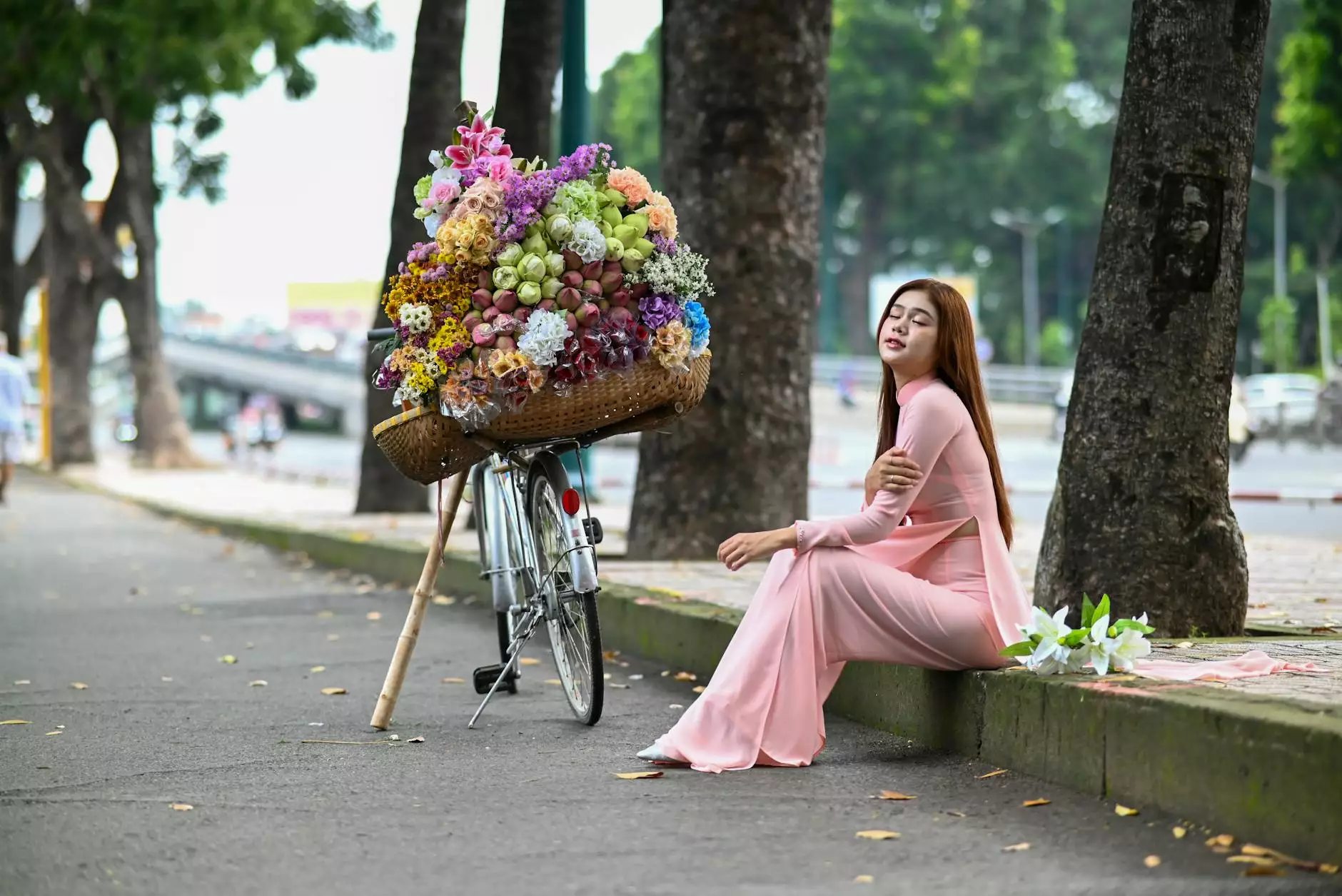
In today's data-driven world, the use of image datasets for classification is more crucial than ever. These datasets empower businesses to harness the full potential of machine learning algorithms, driving better decision-making processes and enhancing product offerings. As a leader in the field, Keylabs.ai provides cutting-edge solutions through our Data Annotation Tool and Data Annotation Platform, ensuring your machine learning models are trained using the most accurate and meaningful data.
Understanding Image Classification
Image classification is a process where a model learns to categorize images into predefined classes. It involves training a machine learning algorithm on a collection of images, which is known as a dataset. This process is foundational for many applications, from facial recognition systems to medical image diagnostics.
The Importance of High-Quality Image Datasets
Quality matters in image datasets. Below are key reasons why high-quality datasets are essential for effective model training:
- Accuracy: High-quality datasets lead to models that can make accurate predictions.
- Diversity: Diverse datasets help the model generalize better across different scenarios.
- Volume: Large volumes of labeled data help avoid overfitting and improve robustness.
Key Components of Image Datasets for Classification
Building an effective image dataset for classification requires attention to several key components:
1. Diversity of Images
A diverse dataset encompasses different backgrounds, lighting conditions, orientations, and scales. For example, a dataset for classifying animals should include images of the same species in various settings, postures, and lighting.
2. Labeled Data
Annotation is the foundation of any dataset. Images need precise labels (e.g., 'cat', 'dog', 'car') to train the model correctly. Our Data Annotation Tool at Keylabs.ai simplifies this process with intuitive features.
3. High Resolution and Quality
Images with high resolution retain more detail, allowing the model to pick up on subtle features that are important for classification. Poor quality images can introduce errors in predictions.
Creating Effective Image Datasets
Creating an image dataset for classification involves a multi-step approach that ensures data quality and relevance:
Step 1: Define Your Objectives
Clearly outline what you aim to achieve with your classification model. Are you detecting objects, identifying faces, or classifying medical images? Your objectives will guide the dataset creation process.
Step 2: Collect Images
Collect images from reliable sources. This may involve:
- Utilizing open-source image repositories.
- Gathering images through web scraping with proper permissions.
- Using company-generated images from relevant contexts.
Step 3: Annotate the Data
Utilize a robust data annotation platform to label your images accurately. Keylabs.ai offers state-of-the-art annotation tools that streamline this process, ensuring high-quality labels.
Step 4: Validate Your Dataset
Before using the dataset for training, validate the annotations. This step is crucial to ensure that the model learns from accurate data, thus reducing the chances of errors during predictions.
Best Practices for Using Image Datasets in Machine Learning
To ensure the success of your classification model, implement these best practices:
1. Continuous Data Updating
Image datasets should not be static. Regularly update your dataset to include new images and labels, ensuring your model adapts to new scenarios and maintains its accuracy over time.
2. Balance Your Dataset
Ensure your dataset is balanced, meaning all classes should have approximately the same number of images. This prevents the model from becoming biased towards classes with more data.
3. Data Augmentation Strategies
Implement image augmentation techniques, such as flipping, rotation, or color adjustment, to artificially increase the size of your dataset. This helps improve the model's ability to generalize.
Real-World Applications of Image Datasets in Classification
The applications of image datasets for classification span multiple industries:
1. Retail and E-commerce
Classifying product images can help enhance the online shopping experience by allowing for better search categorization, personalized recommendations, and inventory management.
2. Healthcare
Medical image classification plays a pivotal role in diagnosing diseases. Datasets consisting of X-ray, MRI, or CT images can help radiologists and AI models in identifying conditions like tumors or fractures.
3. Autonomous Vehicles
In the realm of self-driving cars, image classification datasets are vital. They help vehicles recognize pedestrians, obstacles, and traffic signs to navigate safely.
Challenges in Managing Image Datasets
Despite the advantages, managing image datasets comes with its challenges:
1. Data Privacy
When working with images, especially in sectors like healthcare, adhering to data privacy regulations is paramount. Utilize proper protocols and anonymization techniques when necessary.
2. Annotation Scalability
As datasets grow, the process of annotating becomes increasingly complex. Using automated tools, like the ones offered by Keylabs.ai, can alleviate some of this burden.
3. Handling Imbalanced Datasets
Imbalance in datasets can reduce model performance. Employ strategies like SMOTE (Synthetic Minority Over-sampling Technique) to create balanced datasets, improving overall classification results.
The Future of Image Datasets for Classification
As technology advances, the future of image datasets for classification looks promising:
1. Integration of AI
The integration of AI in dataset creation and annotation processes can enhance efficiency and accuracy. Automated labeling and quality assurance techniques are on the horizon.
2. Collaborative Platforms
The rise of collaborative platforms for sharing and enhancing datasets will further drive innovation in classification tasks. Companies like Keylabs.ai are at the forefront of this evolution, providing tools to facilitate collaboration across teams.
3. Enhanced Anonymization Techniques
With increasing data privacy concerns, the development of advanced anonymization techniques will be vital to protect sensitive information while still allowing for effective dataset usage.
Conclusion
In conclusion, leveraging image datasets for classification is essential for businesses aiming to capitalize on the power of machine learning. At Keylabs.ai, we are committed to providing state-of-the-art Data Annotation Tools and platforms that ensure your datasets are well-defined, accurately labeled, and continuously updated. By understanding the nuances of dataset creation and implementation, organizations can pave the way for successful machine learning initiatives that drive innovation and efficiency.
By prioritizing quality and leveraging advanced tools, you can unlock the true potential of your classification models and stay ahead in the competitive landscape. Let’s embark on this journey towards excellence in machine learning together!