Unlocking Business Potential Through Bayesian Network Analysis
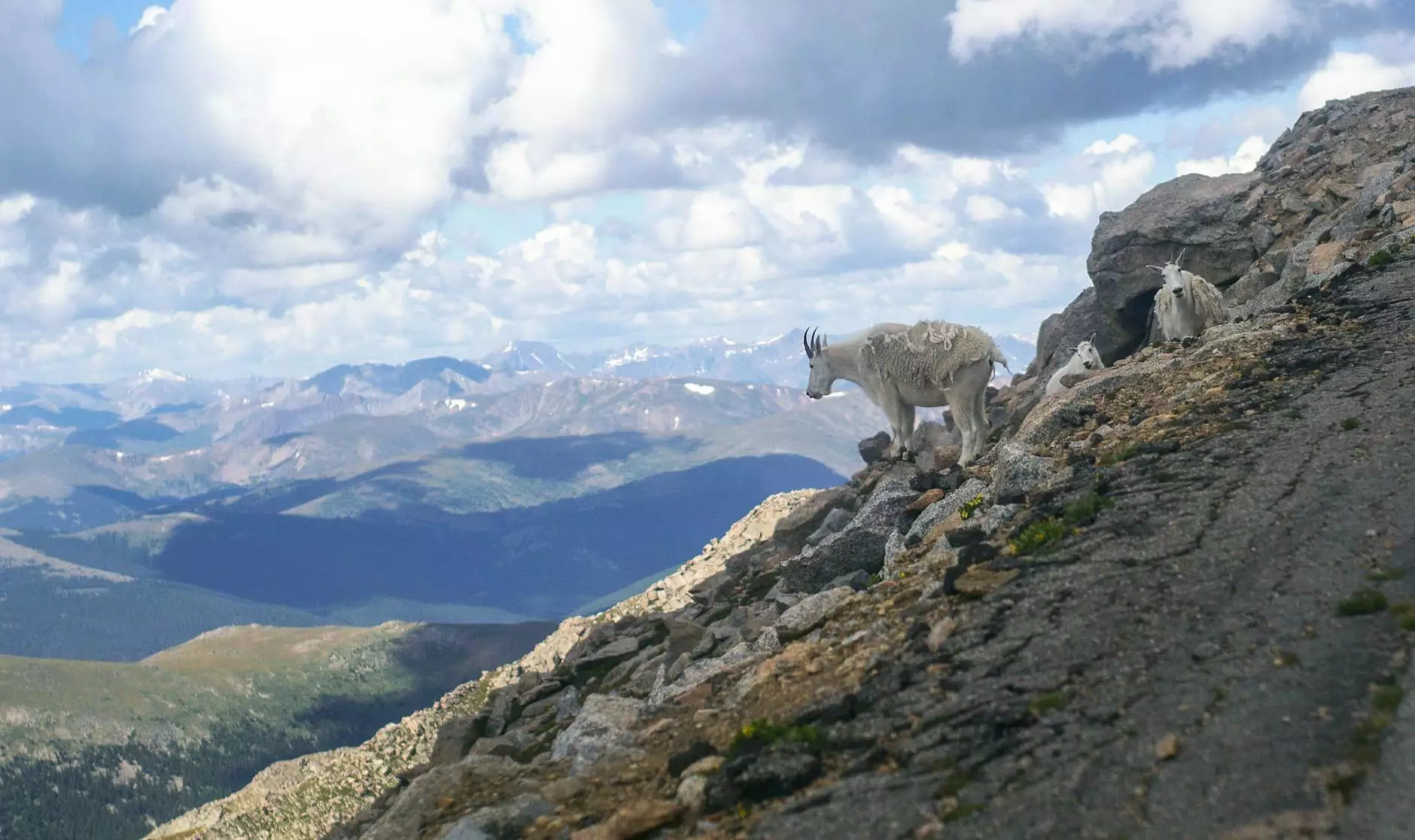
In today's fast-paced digital economy, businesses are constantly seeking ways to leverage data for strategic decision-making. One of the most powerful tools available is Bayesian Network Analysis, a sophisticated method that brings predictive analytics into the business landscape. By analyzing data through probabilistic graphical models, organizations can gain insights that drive growth, enhance security systems, and optimize IT services.
Understanding Bayesian Network Analysis
Bayesian Network Analysis is a statistical technique that uses probability theory to represent and analyze the relationships between variables. At its core, a Bayesian network is a directed acyclic graph (DAG) where nodes represent variables, and edges signify the dependencies between these variables. This framework allows for a comprehensive exploration of complex systems by enabling businesses to make informed decisions based on conditional probabilities.
The Importance of Data in Business
In the realm of business, data is often referred to as the new oil. The ability to extract meaningful insights from vast amounts of information can determine a company's success or failure. Bayesian networks stand out due to their ability to handle ______ uncertainty and variability in data, making them indispensable in various business domains.
Benefits of Using Bayesian Networks in IT Services
For businesses offering IT services and computer repair, incorporating Bayesian Network Analysis can lead to remarkable advantages:
- Improved Fault Detection: By analyzing historical data of system failures, businesses can identify patterns and predict potential failures, allowing for preemptive actions.
- Resource Optimization: Understanding the dependencies between different systems can help IT services optimize resource allocation, reducing costs and increasing efficiency.
- Enhanced Client Support: Predictive analytics can assist in tailoring services to meet the specific needs of clients, thereby improving customer satisfaction.
Bayesian Networks in Security Systems
The integration of Bayesian Network Analysis in security systems has become increasingly critical due to growing concerns about data breaches and cyber threats.
Key Applications in Security
Here are some impactful applications where Bayesian networks enhance security measures:
- Threat Assessment: Bayesian networks can analyze multiple indicators to assess threats to a system, quantifying the likelihood of attacks.
- Incident Response: By predicting the consequences of various security breaches, security teams can devise effective response strategies.
- Tailored Security Protocols: Businesses can use Bayesian analysis to develop security measures that are customized to specific risks, rather than a one-size-fits-all approach.
How Bayesian Network Analysis Works
To effectively implement Bayesian Network Analysis, businesses typically follow several essential steps:
- Model Construction: Identify the key variables that affect the domain and establish the relationships among them.
- Parameter Learning: Using historical data, businesses estimate the probabilities that quantify the relationships between variables.
- Inference: Upon establishing a model, businesses can perform inference to compute the probabilities of unknown variables given known data.
Challenges in Bayesian Network Analysis
While Bayesian Network Analysis offers tremendous benefits, it is not without its challenges. Understanding these aspects can prepare businesses for successful implementation:
Data Challenge
The quality of the insights generated by Bayesian networks heavily depends on the availability of high-quality data. Inaccurate or sparse data can lead to misleading interpretations. Thus, businesses must prioritize data collection and cleansing.
Complexity in Model Building
Building a comprehensive Bayesian model can be complex, particularly when many variables interact in non-linear ways. This complexity necessitates expert knowledge in both the domain of interest and statistical modeling.
Computational Resources
Computing complex Bayesian models can be resource-intensive, requiring substantial processing power. Businesses must ensure they have adequate infrastructure in place.
Implementing Bayesian Network Analysis in Your Business
For businesses interested in harnessing the power of Bayesian Network Analysis, consider the following steps:
Identify Objectives
Every successful implementation begins with a clear understanding of the objectives. What questions do you need your data to answer? What problems are you attempting to solve?
Choose the Right Tools
Invest in the right software tools that facilitate Bayesian analysis. Popular options include:
- BayesiaLab: An intuitive tool designed specifically for building and analyzing Bayesian networks.
- GeNIe: A user-friendly environment for creating graphical decision models, particularly Bayesian networks.
- R Packages: Tools like 'bnlearn' and 'gRain' for model building and probabilistic inference in R.
Gather Your Data
Collect data that encompasses all relevant variables and their interactions. Strive for quality and comprehensiveness to enhance the reliability of the outcome.
Build and Validate Your Model
Create your model based on the gathered data and validate it against known outcomes. Validation is crucial for ensuring accuracy and reliability.
Continuous Improvement
Bayesian networks are not static. They should be continuously refined and improved as new data becomes available, and as conditions change.
The Future of Bayesian Networks in Business
The potential implications of Bayesian Network Analysis are vast and varied, indicating a promising future for its application across numerous industries:
Advancements in AI and Machine Learning
As artificial intelligence (AI) and machine learning technologies continue to evolve, Bayesian networks will likely play an increasingly significant role in automated decision-making processes. The ability of these networks to adapt and learn from new data will enhance their utility in dynamic business environments.
Personalized Customer Experiences
Businesses can utilize Bayesian networks to create personalized experiences for customers based on predictive insights, improving customer engagement and loyalty.
Proactive Risk Management
As organizations face more complex operational risks, the predictive capability of Bayesian networks can lead to proactive risk management strategies, saving time and resources while enhancing operational resilience.
Conclusion
In summary, Bayesian Network Analysis offers a sophisticated blend of statistical rigor and practical application that can empower businesses across various industries. From enhancing IT services and security systems to providing actionable insights for strategic decision-making, the benefits are profound. As we continue through an era defined by data, the organizations that incorporate Bayesian networks into their operational frameworks will be best positioned to lead and succeed.
By adopting this transformative approach, businesses such as Spambrella can remain at the forefront of the IT services market, fostering innovation and resilience over time. Now is the time to embrace the future and unlock your business's full potential through Bayesian Network Analysis.